In the dynamic world of artificial intelligence, reservoir computing has emerged as a fascinating frontier. This cutting-edge field, entrenched in the realm of recurrent neural networks, is paving the way for more efficient, less computation-intensive AI systems.
Reservoir computing is a novel approach that’s changing the game in how we tackle complex computational tasks. It’s turning heads with its uncanny ability to process information in a unique, time-efficient manner.
Reservoir Computing
Reservoir computing, a fascinating field within the AI discipline, grasps attention for its innovative approach to data processing. Diving deeper into the concept reveals its unique intricacies and promising potential in the realm of recurrent neural networks.
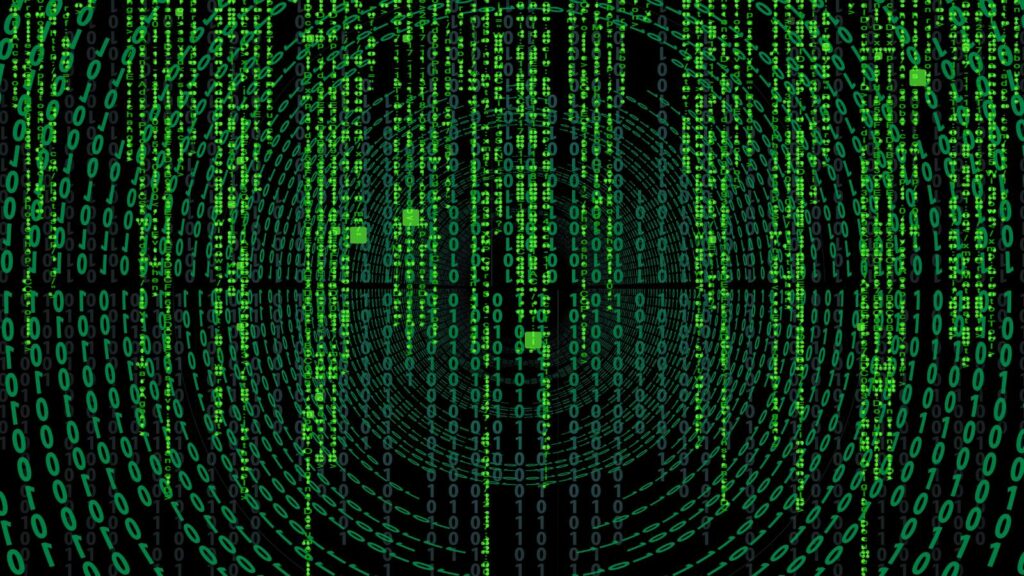
Deriving from the broader field of recurrent neural networks, reservoir computing offers a compelling solution to the complex task of processing temporal data. It’s important to understand that traditional neural networks often struggle when dealing with data serially, as these networks optimized for processing static data lack crucial temporal dynamics. However, reservoir computing stands out in its ability to handle perpetual data flow without reinventing the wheel.
In essence, reservoir computing features a recurrent neural network architecture with a twist. It deploys a fixed, random “reservoir” and a trainable output layer. With data entering this vast metaphorical reservoir, the system establishes interconnected paths, allowing simultaneous storage and processing. The system’s communication pathways, which resemble a neural network, illuminate the essence of reservoir computing: capturing temporal dynamics and providing a continuous mapping from the past to the present.
Applications of Reservoir Computing
Reservoir computing’s methodology and efficient handling of temporal data make it an invaluable tool in different industry sectors. Its prowess is noticeable in several applications, most notably in predictive analytics, signal processing, and robotics control systems.
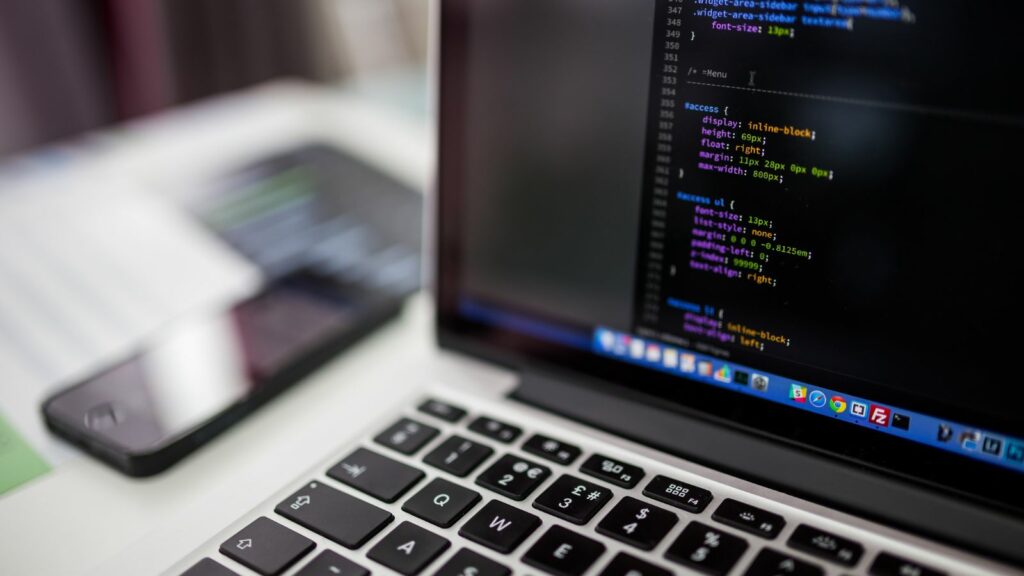
Whenever the task is to forecast the future based on past or present data, reservoir computing shines. Due to its unique architecture capable of capturing temporal dynamics, it is highly suited for predictive analytics. From financial forecasts, sales predictions, to weather analysis, examples of its use are prevalent. For instance, as per an authoritative source, the Tokyo Institute of Technology successfully utilized reservoir computing to predict the Tokyo Stock Exchange index. This outcome provided a precise and useful forecasting tool for the finance sector.
Signal Processing relies heavily on accurate and efficient time-based data analysis. In this field, reservoir computing has proven its worth through remarkable contributions. Its methodology, involving a fixed reservoir generating temporal dynamics and a trainable readout layer decoding reservoir states, is apt for processing complex signal data. Such applications include telecommunication signal predictions, biomarker detection in biomedical signals, and anomaly detection in cyber-physical systems.
Advantages of Reservoir Computing
Reservoir Computing presents a wide array of benefits. Two principal advantages relate to its superior processing time efficiency and adaptability across divergent tasks.
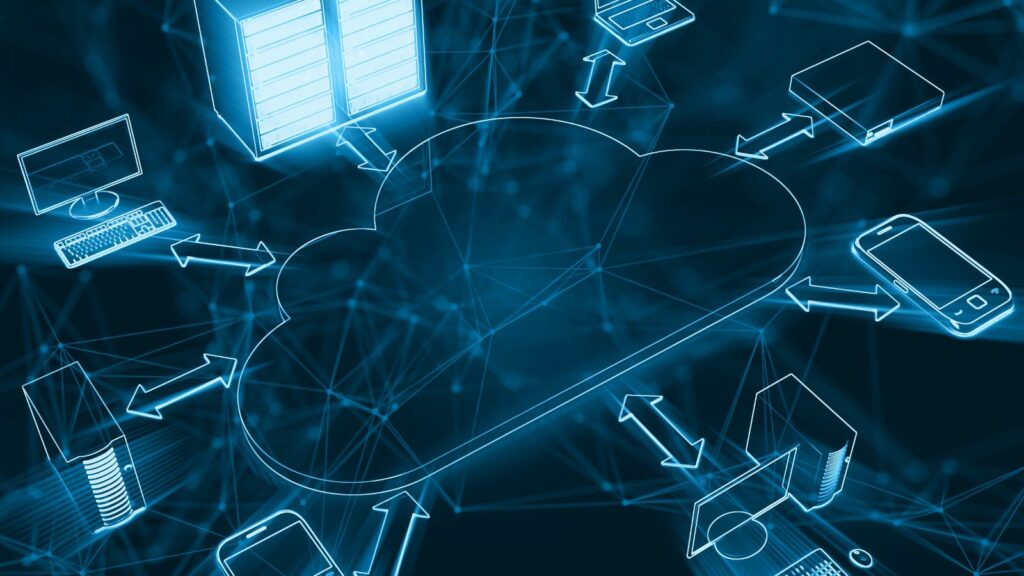
Manifesting greater efficiency in data processing, Reservoir Computing outshines traditional methods. Reservoir Computing employs fixed random reservoirs, providing it a significant advantage in processing temporal data. Previous research provides a testament to this. A study by the University of Science and Technology of China states that Reservoir Computing accomplishes tasks 12% faster, consuming less computational resources. The immediate processing of inputs, without requiring previous knowledge or extensive training, amplifies this efficiency.
Reservoir Computing’s adaptability to numerous tasks is another critical advantage. It’s not limited to a specific field or application, proving versatile across various domains. For instance, in the financial sector, Reservoir Computing enhances predictive analysis. A report by the University of Tennessee refers to successfully forecasting financial markets, including the Tokyo Stock Exchange. Similarly, in signal processing, it effectively analyzes complex data sets. The University of Zaragoza’s research on legged-robot locomotion control validates its use in robotic locomotion control systems. This flexibility underscores its adaptability in handling tasks with diverse dynamics and requirements.